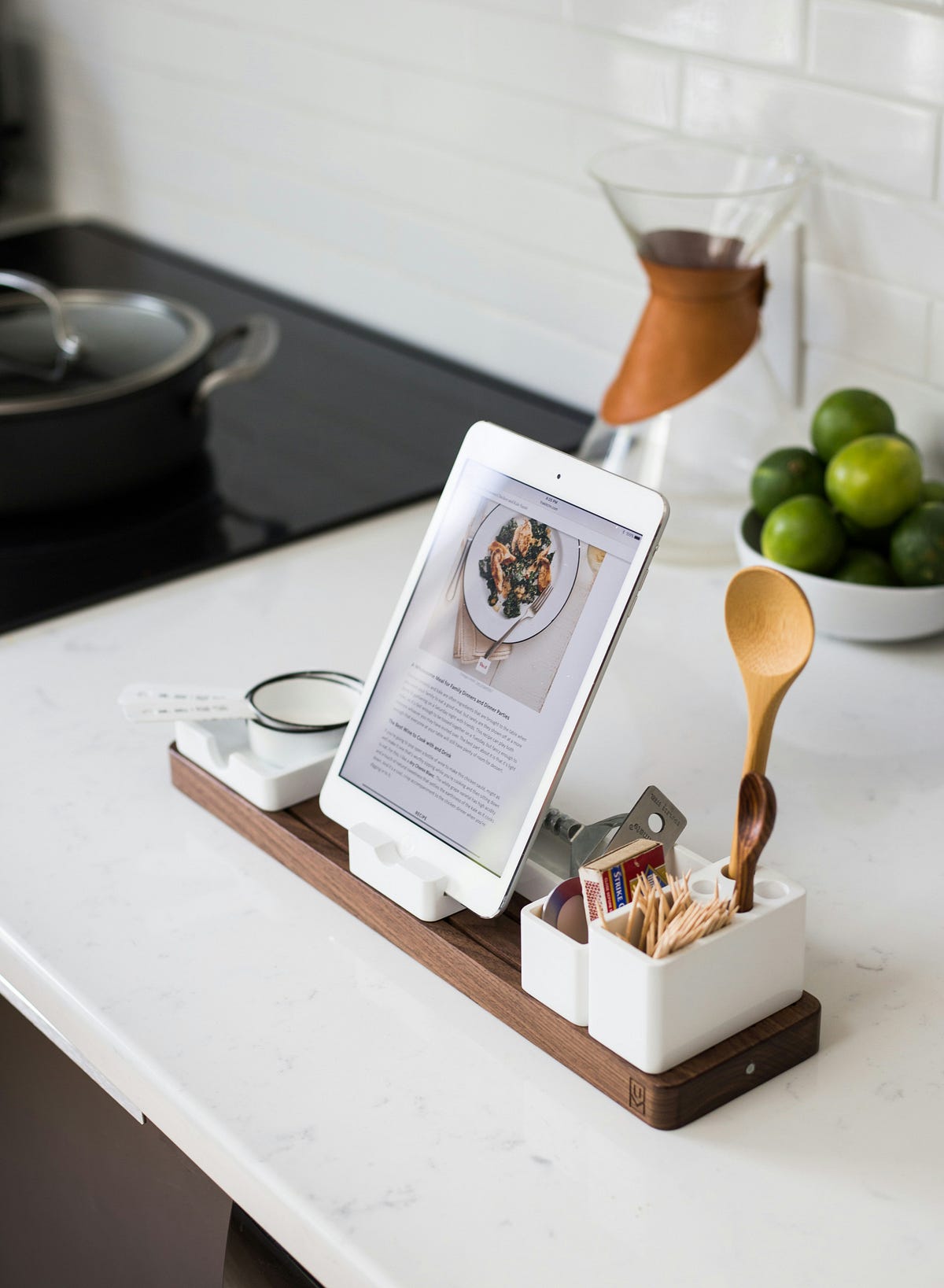
Di recente, stavo cercando un set di dati di ricette open source per un progetto personale, ma non sono riuscito a trovarne nessuno tranne questo repository github contenente le ricette visualizzate su publicdomainrecipes.com.
Sfortunatamente, avevo bisogno di un set di dati che fosse più sfruttabile, cioè qualcosa di più vicino a dati tabellari o ad a Documento NoSQL. È così che ho pensato di trovare un modo per trasformare i dati grezzi in qualcosa di più adatto alle mie esigenze, senza spendere ore, giorni e settimane a farlo manualmente.
Lascia che ti mostri come ho utilizzato la potenza dei modelli linguistici di grandi dimensioni per automatizzare il processo di conversione del testo non elaborato in documenti strutturati.
Set di dati
Il set di dati originale è una raccolta di file di markdown. Ogni file rappresenta una ricetta.
Come puoi vedere, questo non è completamente destrutturato, ci sono dei bei metadati tabulari nella parte superiore del file, quindi ci sono 4 sezioni distinte:
- Un introduzione,
- L'elenco degli ingredienti
- Indicazioni
- Alcuni suggerimenti.
Sulla base di questa osservazione, Sebastiano Bahrsviluppato un parser per trasformare i file markdown in JSON Qui.
L'output del parser è già più sfruttabile, oltretutto Sebastian lo usava costruire un chatbot per consigliare ricette. Tuttavia, ci sono ancora alcuni inconvenienti. I tasti ingredienti e indicazioni contengono testi grezzi che potrebbero essere meglio strutturati.
Così com'è, alcune informazioni utili sono nascoste.
Ad esempio, le quantità degli ingredienti, il tempo di preparazione o di cottura per ogni passaggio.
Codice
Nel resto di questo articolo, mostrerò i passaggi che ho intrapreso per ottenere documenti JSON simili a quello seguente.
{
"name": "Crêpes",
"serving_size": 4,
"ingredients": (
{
"id": 1,
"name": "white flour",
"quantity": 300.0,
"unit": "g"
},
{
"id": 2,
"name": "eggs",
"quantity": 3.0,
"unit": "unit"
},
{
"id": 3,
"name": "milk",
"quantity": 60.0,
"unit": "cl"
},
{
"id": 4,
"name": "beer",
"quantity": 20.0,
"unit": "cl"
},
{
"id": 5,
"name": "butter",
"quantity": 30.0,
"unit": "g"
}
),
"steps": (
{
"number": 1,
"description": "Mix flour, eggs, and melted butter in a bowl.",
"preparation_time": null,
"cooking_time": null,
"used_ingredients": (1,2,5)
},
{
"number": 2,
"description": "Slowly add milk and beer until the dough becomes fluid enough.",
"preparation_time": 5,
"cooking_time": null,
"used_ingredients": (3,4)
},
{
"number": 3,
"description": "Let the dough rest for one hour.",
"preparation_time": 60,
"cooking_time": null,
"used_ingredients": ()
},
{
"number": 4,
"description": "Cook the crêpe in a flat pan, one ladle at a time.",
"preparation_time": 10,
"cooking_time": null,
"used_ingredients": ()
}
)
}
Il codice per riprodurre il tutorial è su GitHub Qui.
Mi sono affidato a due potenti librerie langchain
per comunicare con i fornitori LLM e pydantic
per formattare l'output degli LLM.
Per prima cosa ho definito i due componenti principali di una ricetta con il file Ingredient
E Step
classi.
In ogni lezione ho definito gli attributi rilevanti e fornito una descrizione del campo ed esempi. Questi vengono poi forniti ai LLM da langchain
portando a risultati migliori.
"""`schemas.py`"""from pydantic import BaseModel, Field, field_validator
class Ingredient(BaseModel):
"""Ingredient schema"""
id: int = Field(
description="Randomly generated unique identifier of the ingredient",
examples=(1, 2, 3, 4, 5, 6),
)
name: str = Field(
description="The name of the ingredient",
examples=("flour", "sugar", "salt")
)
quantity: float | None = Field(
None,
description="The quantity of the ingredient",
examples=(200, 4, 0.5, 1, 1, 1),
)
unit: str | None = Field(
None,
description="The unit in which the quantity is specified",
examples=("ml", "unit", "l", "unit", "teaspoon", "tablespoon"),
)
@field_validator("quantity", mode="before")
def parse_quantity(cls, value: float | int | str | None):
"""Converts the quantity to a float if it is not already one"""
if isinstance(value, str):
try:
value = float(value)
except ValueError:
try:
value = eval(value)
except Exception as e:
print(e)
pass
return value
class Step(BaseModel):
number: int | None = Field(
None,
description="The position of the step in the recipe",
examples=(1, 2, 3, 4, 5, 6),
)
description: str = Field(
description="The action that needs to be performed during that step",
examples=(
"Preheat the oven to 180°C",
"Mix the flour and sugar in a bowl",
"Add the eggs and mix well",
"Pour the batter into a greased cake tin",
"Bake for 30 minutes",
"Let the cake cool down before serving",
),
)
preparation_time: int | None = Field(
None,
description="The preparation time mentioned in the step description if any.",
examples=(5, 10, 15, 20, 25, 30),
)
cooking_time: int | None = Field(
None,
description="The cooking time mentioned in the step description if any.",
examples=(5, 10, 15, 20, 25, 30),
)
used_ingredients: list(int) = Field(
(),
description="The list of ingredient ids used in the step",
examples=((1, 2), (3, 4), (5, 6), (7, 8), (9, 10), (11, 12)),
)
class Recipe(BaseModel):
"""Recipe schema"""
name: str = Field(
description="The name of the recipe",
examples=(
"Chocolate Cake",
"Apple Pie",
"Pasta Carbonara",
"Pumpkin Soup",
"Chili con Carne",
),
)
serving_size: int | None = Field(
None,
description="The number of servings the recipe makes",
examples=(1, 2, 4, 6, 8, 10),
)
ingredients: list(Ingredient) = ()
steps: list(Step) = ()
total_preparation_time: int | None = Field(
None,
description="The total preparation time for the recipe",
examples=(5, 10, 15, 20, 25, 30),
)
total_cooking_time: int | None = Field(
None,
description="The total cooking time for the recipe",
examples=(5, 10, 15, 20, 25, 30),
)
comments: list(str) = ()
Dettagli tecnici
- È importante non avere un modello troppo rigido in questo caso, altrimenti la convalida pydantic del JSON emesso da LLM fallirà. Un buon modo per dare una certa flessibilità è anche fornire valori predefiniti come
None
o elenchi vuoti()
a seconda del tipo di output target. - Notare la
field_validator
sulquantity
attributo delIngredient
è lì per aiutare il motore ad analizzare le quantità. Inizialmente non era presente, ma facendo alcune prove, ho scoperto che LLM spesso forniva quantità come stringhe come1/3
O1/2
. - IL
used_ingredients
permettono di collegare formalmente gli ingredienti ai passaggi rilevanti delle ricette.
Il modello dell'output in fase di definizione del resto del processo è piuttosto fluido.
In un prompt.py
file, ho definito a create_prompt
funzione per generare facilmente prompt. Per ogni ricetta viene generato un “nuovo” prompt. Tutti i prompt hanno la stessa base ma la ricetta stessa viene passata come variabile al prompt di base per crearne una nuova.
""" `prompt.py`The import statements and the create_prompt function have not been included
in this snippet.
"""
# Note : Extra spaces have been included here for readability.
DEFAULT_BASE_PROMPT = """
What are the ingredients and their associated quantities
as well as the steps to make the recipe described
by the following {ingredients} and {steps} provided as raw text ?
In particular, please provide the following information:
- The name of the recipe
- The serving size
- The ingredients and their associated quantities
- The steps to make the recipe and in particular, the duration of each step
- The total duration of the recipe broken
down into preparation, cooking and waiting time.
The totals must be consistent with the sum of the durations of the steps.
- Any additional comments
{format_instructions}
Make sure to provide a valid and well-formatted JSON.
"""
La comunicazione con la logica LLM è stata definita nelrun
funzione delcore.py
file, che non mostrerò qui per brevità.
Alla fine, ho combinato tutti questi componenti nel mio filedemo.ipynb
taccuino il cui contenuto è mostrato di seguito.
# demo.ipynb
import os
from pathlib import Pathimport pandas as pd
from langchain.output_parsers import PydanticOutputParser
from langchain_mistralai.chat_models import ChatMistralAI
from dotenv import load_dotenv
from core import run
from prompt import DEFAULT_BASE_PROMPT, create_prompt
from schemas import Recipe
# End of first cell
# Setup environment
load_dotenv()
MISTRAL_API_KEY = os.getenv("MISTRAL_API_KEY") #1
# End of second cell
# Load the data
path_to_data = Path(os.getcwd()) / "data" / "input" #2
df = pd.read_json("data/input/recipes_v1.json")
df.head()
# End of third cell
# Preparing the components of the system
llm = ChatMistralAI(api_key=MISTRAL_API_KEY, model_name="open-mixtral-8x7b")
parser = PydanticOutputParser(pydantic_object=Recipe)
prompt = create_prompt(
DEFAULT_BASE_PROMPT,
parser,
df("ingredients")(0),
df("direction")(0)
)
#prompt
# End of fourth cell
# Combining the components
example = await run(llm, prompt, parser)
#example
# End of fifth cell
ero solito Mistral come fornitore LLM, con il loro open-mixtral-8x7b
modello che rappresenta un'ottima alternativa open source a OpenAI. langchain
ti consente di cambiare facilmente fornitore se hai creato un account sulla piattaforma del fornitore.
Se stai cercando di riprodurre i risultati:
- (#1) – Assicurati di avere a
MISTRAL_API_KEY
in un file .env o nell'ambiente del sistema operativo. - (#2) – Fai attenzione al percorso dei dati. Se cloni il mio repository, questo non sarà un problema.
L'esecuzione del codice sull'intero set di dati costa meno di 2€.
È possibile trovare il set di dati strutturati risultante da questo codice Qui nel mio archivio.
Sono soddisfatto dei risultati, ma potrei comunque provare a ripetere il prompt, le descrizioni dei miei campi o il modello utilizzato per migliorarli. Potrei provare il modello più recente di MistralAI, il open-mixtral-8x22b
oppure provare un altro provider LLM semplicemente modificando 2 o 3 righe di codice grazie a langchain
.
Quando sarò pronto, potrò tornare al mio progetto originale. Restate sintonizzati se volete sapere di cosa si trattava. Nel frattempo, fammi sapere nei commenti cosa faresti con il set di dati finale?
I Large Language Models (LLM) offrono un potente strumento per strutturare dati non strutturati. La loro capacità di comprendere e interpretare le sfumature del linguaggio umano, automatizzare attività laboriose e adattarsi all'evoluzione dei dati li rende una risorsa inestimabile nell'analisi dei dati. Sbloccando il potenziale nascosto all’interno dei dati testuali non strutturati, le aziende possono trasformare questi dati in informazioni preziose, favorendo un migliore processo decisionale e risultati aziendali. L'esempio fornito, della trasformazione dei dati grezzi delle ricette in un formato strutturato, è solo una delle innumerevoli possibilità offerte dai LLM.
Mentre continuiamo a esplorare e sviluppare questi modelli, possiamo aspettarci di vedere molte altre applicazioni innovative in futuro. Il viaggio per sfruttare tutto il potenziale degli LLM è appena iniziato e la strada da percorrere promette di essere entusiasmante.
Fonte: towardsdatascience.com