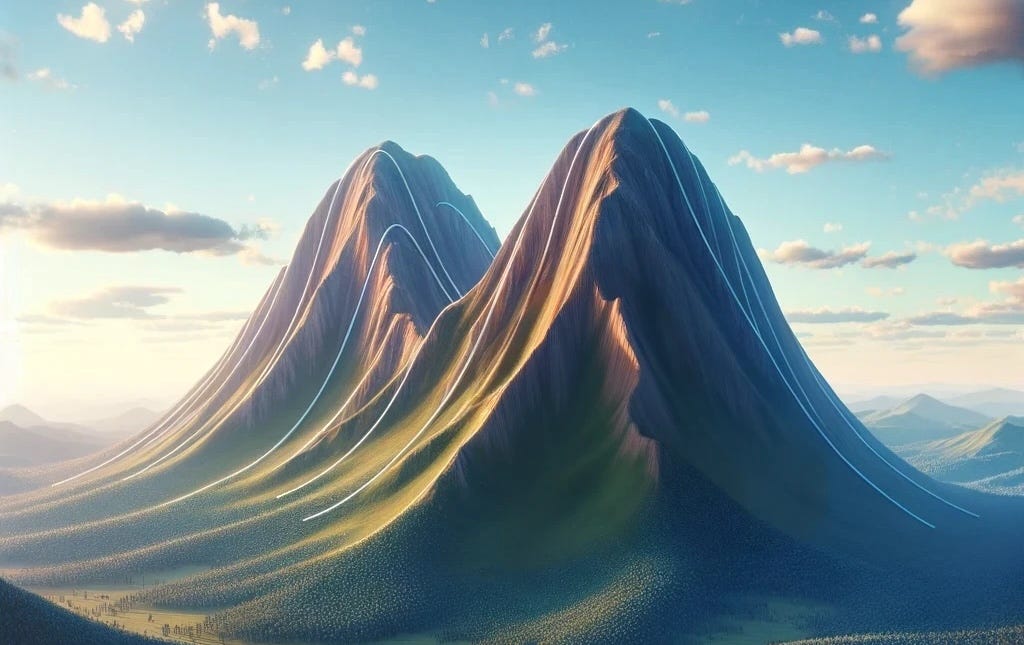
Un metodo di ottimizzazione numerica popolare e ad alte prestazioni è Il metodo di Brent. Il metodo di Brent è un algoritmo per la ricerca delle radici che combina varie tecniche come il metodo della bisezione, il metodo delle secanti e l'interpolazione quadratica inversa. Ulteriori dettagli sulla sua implementazione possono essere trovati in Statsmodels Qui.
In Python, l'implementazione è simile alla seguente:
def solve_power(self, effect_size=None, nobs1=None, alpha=None, power=None,
ratio=1., alternative='two-sided'):
print('--- Arguments: ---')
print('effect_size:', effect_size, 'nobs1:', nobs1, 'alpha:', alpha, 'power:', power, 'ratio:', ratio, 'alternative:', alternative, '\n')# Check that only nobs1 is None
kwds = dict(effect_size=effect_size, nobs1=nobs1, alpha=alpha,
power=power, ratio=ratio, alternative=alternative)
key = (k for k,v in kwds.items() if v is None)
assert(key == ('nobs1'))
# Check that the effect_size is not 0
if kwds('effect_size') == 0:
raise ValueError('Cannot detect an effect-size of 0. Try changing your effect-size.')
# Initialize the counter
self._counter = 0
# Define the function that we want to find the root of
# We want to find nobs1 s.t. current power = target power, i.e. current power - target power = 0
# So func = current power - target power
def func(x):
kwds('nobs1') = x
target_power = kwds.pop('power') # always the same target power specified in keywords, e.g. 0.8
current_power = self.power(**kwds) # current power given the current nobs1, note that self.power does not have power as an argument
kwds('power') = target_power # add back power to kwds
fval = current_power - target_power
print(f'Iteration {self._counter}: nobs1 = {x}, current power - target power = {fval}')
self._counter += 1
return fval
# Get the starting values for nobs1, given the brentq_expanding algorithm
# In the original code, this is the self.start_bqexp dictionary set up in the __init__ method
bqexp_fit_kwds = {'low': 2., 'start_upp': 50.}
# Solve for nobs1 using brentq_expanding
print('--- Solving for optimal nobs1: ---')
val, _ = brentq_expanding(func, full_output=True, **bqexp_fit_kwds)
return val
1.2. Scrivere una versione ridotta di tt_ind_solve_power che sia un'implementazione esatta della derivazione statistica e produca lo stesso output della funzione originale
Il file sorgente in Statsmodels è disponibile Qui. Sebbene la funzione originale sia scritta per essere più potente, la sua generalizzabilità rende anche più difficile ottenere intuizioni su come funziona il codice.
Ho quindi esaminato il codice sorgente riga per riga e l'ho semplificato da 1.600 righe di codice a 160 e da oltre 10 funzioni a solo 2, assicurandomi al tempo stesso che l'implementazione rimanesse identica.
Il codice ridotto contiene solo due funzioni nella classe TTestIndPower, seguendo esattamente la derivazione statistica spiegata nella Parte 1:
- energiache calcola la potenza data una dimensione del campione
- risolvere_potereche trova la dimensione minima del campione che raggiunge una potenza target utilizzando il metodo di Brent
Questo è il codice completo per la versione ridotta con un test per verificare che produca lo stesso output della funzione originale:
Fonte: towardsdatascience.com